Today’s AI algorithms provide medical recommendations by analyzing big data, but they can’t always give a reason for their conclusions other than the patterns they detect. Even though these AI-recommended solutions can’t be explained in terms of human understanding, many such treatments might improve the quality of patients’ lives and even save lives. This article discusses the controversial topic of medical explainability from a viewpoint that supports applying technological advancements to healthcare.
Introduction
It’s no secret that the AI revolution has begun. I’m not the only one who believes that AI is making significant changes to our world. These quotes from some of the best-known leaders in science and technology point in the same direction:
Success in creating AI would be the biggest event in human history. Unfortunately, it might also be the last, unless we learn how to avoid the risks.
— Stephen Hawking, Theoretical Physicist
. . .
What all of us have to do is to make sure we are using AI in a way that is for the benefit of humanity, not to the detriment of humanity.–
— Tim Cook, Apple CEO
. . .
Some people call this artificial intelligence, but the reality is this technology will enhance us. So instead of artificial intelligence, I think we’ll augment our intelligence.
— Ginni Rometty, IBM CEO
. . .
AI is one of the most important things humanity is working on. It is more profound than, I dunno, electricity or fire.
— Sundar Pichai, Google CEO
. . .
As some of the most brilliant minds on earth have said, it isn’t just hype.
AI is a tectonic movement, backed by the most powerful companies in the world.
Even if we don’t notice, we use its applications every single day.
. . .
The healthcare industry
I have a problem when I see the word healthcare next to industry. It seems like we’re talking about a basic human right next to a word that means a sellable product. This industry relies heavily on human intervention and subjective opinions. It uses advanced technologies like genome decoding, MRIs, PET scans, and radiotherapy., But it also strongly depends on human interpretations, and humans make mistakes.
AI and machine learning applications can deliver extreme benefits to healthcare service. No doctor in the world can analyze millions of cases or cross-reference treatment responses with genome analysis in a fraction of a second. Machine learning has those capabilities and more, and the medical community knows that. But the ecosystem — from small-town doctors to top Ivy League researchers — is highly resistant to change. This resistance isn’t founded on reality. It’s based on professional bias and fear.
The broken U.S. healthcare system
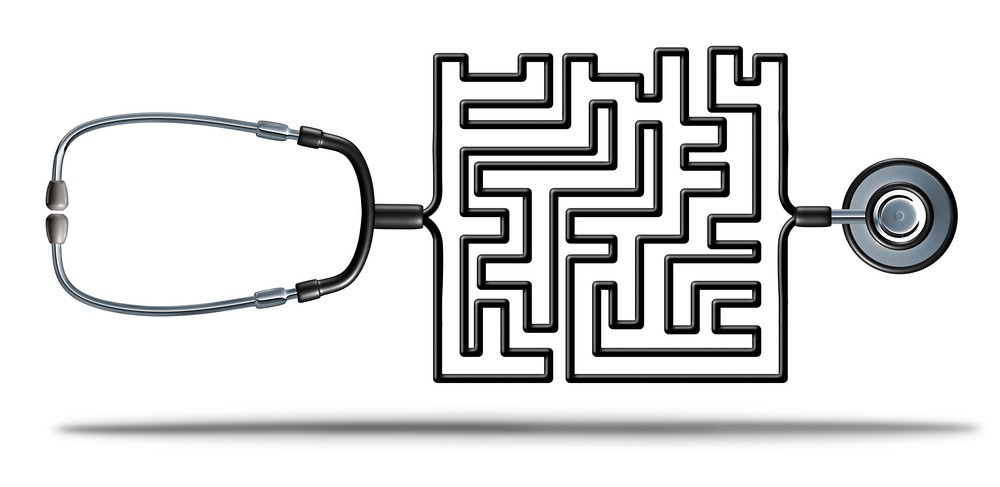
For those of us who were born in other countries where the best healthcare is freely available to everyone, America’s healthcare system seems insane. To be clear, I’m not an expert. But one famous example is when former Vice President of the U.S. Joe Biden couldn’t afford his son’s cancer treatment, and Barack Obama offered him a loan. That situation makes me think that something is very wrong. At the time, Biden even considered selling his home. He was the Vice President of the United States. If he can’t afford healthcare, how can people like middle-class Americans from Alabama and people with even lower incomes afford it?
Insane prices for simple diseases, overpriced patented medications, and an expensive insurance system with restricted access all add up to a difficult situation. To cope, many Americans resort to healthcare tourism. They can get state-of-the-art treatments abroad for a fraction of what they’d cost in the U.S.
Resistance to change
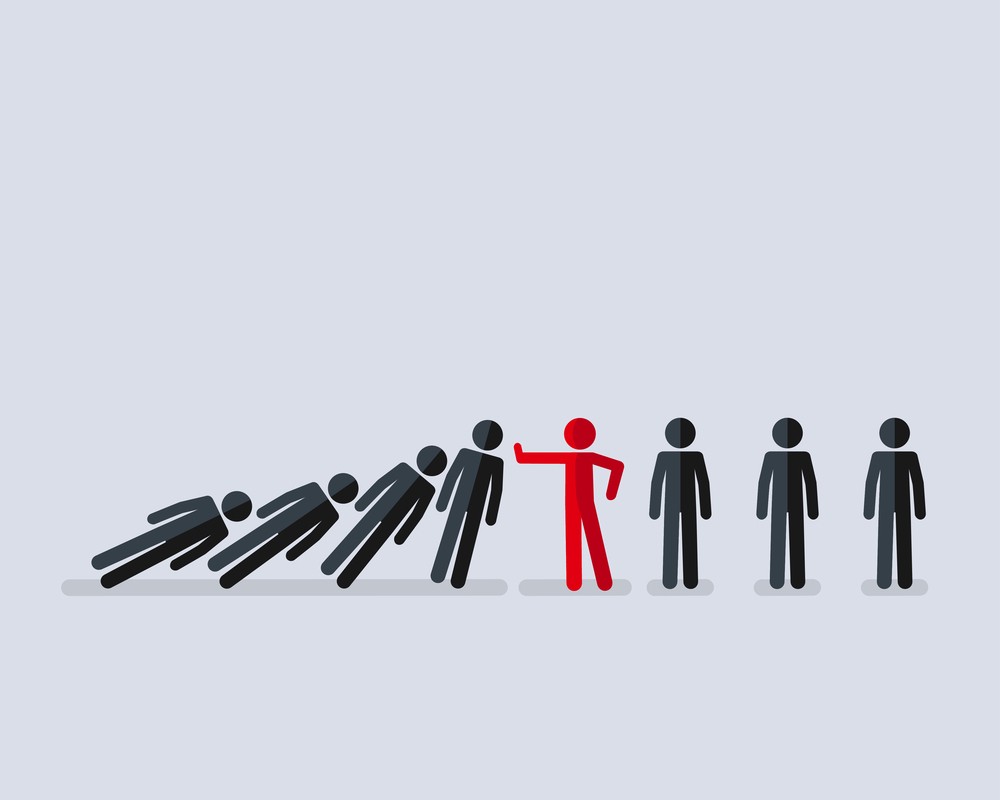
I once heard that the U.S. has the best healthcare system in the world if you can pay for it.I believe that it’s the most expensive, but I doubt it’s the best. One problem might be physicians. Don’t kill the messenger, but doctors make more money in the U.S. than in most other countries, and that’s part of the problem. Of course, one of the country’s highest-paying professions will appeal to people who are motivated by financial gain. In some cases, improving the lives of others might be less of an incentive than a big salary in the decision to pursue a career in medicine.
Economics and AI
Naturally, those who practice medicine for its monetary benefits won’t want to lose that income, even if healthcare would advance as a result of that loss. As an example, AI has the potential to gradually augment or replace doctors. If you go to a medical congress, only a small percentage of the presentations demonstrate some new kind of AI or machine learning technology application. The U.S. medical community is hesitant to adopt these advances, even if they might vastly improve the overall health of people in the U.S.
Another problem: researchers

This issue relates to the previous section about doctors. Most medical research is seriously flawed. From the mathematics and statistical point of view, there are humongous problems. One such problem is the confusion between correlation and causation. An example is a children’s music lesson experiment where statistically simple correlations were found, and researchers concluded that one thing caused another. If medical researchers need to come up with conclusions to get their papers approved and thereby get continued funding, they might resort to that type of sloppy research practices.
Another huge topic is who finances medical research. In many cases, research investors are enterprises with a strong interest in making a profit, but I won’t dive deep into that here.
Conflicting conclusions
In any case, some of the most important medical papers are inconclusive or flawed, and we don’t have many certainties:
- Is cholesterol good or bad? What about LDL?
- Is meat good or bad? Do vegetarians have better health?
- Is dairy bad for your health?
- Does cheese cause cancer?
- Should you follow the Paleo or Keto diets?
- Should you eat low-fat food? Or low carb?
If you check medical research on these topics, you might find contradictory studies that point in completely opposite directions. That can happen when a correlation is mistaken for causation.
Observational evidence
One big problem is that so much medical research is based on observational evidence, and the medical community supports it as a valid method. An example is observational nutritional epidemiology, which tracks people’s nutritional habits and their diseases. But there are many variables in that field of medicine: conflicts of interest, different lifestyles, and lack of mathematical and statistical foundations. Most observational studies are subjective, and by nature, subjective conclusions are biased. You decide what to believe or not based on your personal belief systems, but your decision isn’t based on scientifically valid evidence.
A possible solution: How the body works
To overcome the limitations of observational research, we can explore deeper by studying the mechanisms that cause different things to happen, like these examples:
- How meat interacts with cells.
- How fat is burnt in the brain.
- How cancer develops.
- How arteries deteriorate.
This type of research is more complex than mere subjective observation by many orders of magnitude. It implies studying the mechanisms or chemical transformations and interactions between our smallest living units. Are we ready for that? Are we ready for a whole new human chemistry model? Probably not yet.
At present, the forces that shape our society and economic systems are too complex to permit drastic changes from the status quo. But hopefully, we won’t have to wait a few centuries to experience any measurable advances in healthcare. Although this approach might lead to important breakthroughs and shouldn’t be underestimated, it’s not a practical solution at this time. But there are other possible solutions that involve AI and machine learning.
Deep learning and big data
Thanks to today’s advances in computer technology, we can process data points in ways beyond what we could ever imagine in the past. We can analyze the human genome, find correlations, conjecture causation, and check millions of medical causes and outcomes.
With deep learning or neural networks, we can find the best treatment for each person, save lives, and improve the quality of life for millions of people. This machine learning application can treat people in remote places of the world and prescribe better treatments than even the best human doctors. Augmenting doctors’ capacity with AI advice, just like a second opinion, might be a game-changer in healthcare.
A real-life application

I was recently involved in a cancer research study where doctors couldn’t distinguish with imageology if the cancer cells were malign or benign. Chemotherapy followed by an invasive surgery to find out whether the patient responded to chemo is a standard treatment. Based on data analysis of thousands of cancer cases, deep learning can analyze imageology exams and determine if a particular patient will respond to chemo or not. In this way, AI can help a patient avoid unnecessary chemo and surgery, improving life quality and expectancy.
Banned treatments
Although this life-enhancing medical technology exists, it’s not available to cancer patients. That’s because the FDA won’t approve treatments that aren’t explainable. Deep learning algorithms usually make the right decision, and we can measure that. AIs are designed to work like our brains. But they’re more like our brain on steroids. By analyzing complex data far beyond anything our brains are capable of processing, machines detect patterns and predict behavior. But they can’t explain their decisions in a way we can understand.
A caveat: we’re much more error-tolerant with humans than with machines
Before we dive into the explainability dilemma, let’s talk about the mistakes made by humans. We’re much more comfortable with human error than we are with machine error. An example of that is self-driving cars. According to the company’s report for Q4 2019, the Tesla Autopilot travels six times farther without accidents than self-driven cars. However, whenever there’s a crash on Autopilot, even one that would have also been unavoidable by a human driver, people are quick to demand bans on self-driving cars. In a recent case, a lobbyist represented taxi and limo drivers who would financially benefit from their absence.
A comparative example: transportation deaths
About 1.5 million people in the world die in traffic accidents every year. This statistic equates to 23 Boeing 737–800s crashing every day with no survivors. No one would tolerate this level of danger from airplanes, and computer systems would likely take the blame. But if autonomous cars had just 1 percent of this number of casualties or about 15,000 deaths per year, some people go nuts over this technology.
The big dilemma: Explainability

What if we can make optimal decisions about how to treat diseases, but we can’t explain how we made them? What if our decisions are based on an analysis of millions of cases, but we can’t say why or what factors were the most relevant? This problem is the explainability dilemma.
Explainable AI
One solution might be explainable AI (XAI), which refers to methods and techniques in the application of AI technology with results that can be understood by human experts. It contrasts with the concept of the black boxin machine learning, where even the designers can’t explain why their AI arrived at a specific decision. In the future, XAI might be the pathway for many machine learning-recommended healthcare treatments to get approved. But unfortunately, XAI’s decision-making power is vastly limited compared to non-explainable AI.
Make it personal
At some point in your life, you’ll probably get some type of medical treatment. Now consider these questions:
- If you get cancer, do you want the most optimal treatment, even if you couldn’t understand why or how an AI system came up with that recommendation?
- Or do you prefer a sub-optimal treatment because you can understand why your doctor recommends the treatment?
Explainability and the FDA
In the future, there will likely be consequences if the FDA continues to reject treatments that aren’t explainable. People might see others benefiting from AI-recommended treatments in less regulated countries. And this evidence might incentivize the healthcare tourism industry to grow exponentially if the same treatments continue to be unavailable in the U.S.
Limits of explainable approaches
Can we achieve a research process that is both optimal and explainable? That seems unlikely because of the nature of machine learning. It’s designed by humans to mimic human brain function, but machine learning goes beyond anything a human brain can do or understand. AI tech is becoming more complex as it advances, but our brains can’t evolve in the same way as the technology we create.
Resistance to change
Doctors and investors who benefit financially from things staying the same might resist change to the current FDA regulations that apply to AI. And change can also be frightening to patients and social collectives that look to the medical community for expert guidance. But changes have a way of happening despite our fears.
A possible future

Will the future of medicine be the lack of explainability? In some ways, that’s a scary thought, but it might be true. The advancement of XAI can help bridge the gap of understanding, but it will require investments and time.
At some point, will we let the results speak for themselves?
If so, we’ll need to create a whole new ethical framework and prepare for big changes.
Unstoppable science

Throughout human history, people have resisted new scientific ideas that challenged their belief systems. But despite that resistance, scientific knowledge and technology continue to evolve. And we continue to reap the benefits.
The advancement of medical technology through AI will likely be unstoppable as well.
Hopefully, physicians and the healthcare industry won’t be more of a problem than a solution.