Handling data is a big part of developing applications with Django. To do that, we use Django's ORM, from retrieving a specific set of instances to updating several rows in the database.
It's no surprise then that the way we make those queries can significantly impact our app's performance. So, in this blog post, we will look into some valuable functionalities provided by Django.
We will also provide some best practices to keep in mind when writing our queries and how we can combine them to maximize the efficiency of our application.
Django ORM and Querysets
A queryset is a collection of data from the database. The Django ORM allows us to easily make queries in Python without the need of using the SQL language.
Querysets are lazy, meaning that just creating a queryset does not trigger any database hits. You can add filters and modify the same queryset many times, but it will only access the database once it needs to be evaluated.
CODE: https://gist.github.com/brunomichetti/d0d5430bc8b0181acdb2ea0a7fa103f3.js
In this example, even though we create and manipulate the queryset more than once, the only time when Django accesses the database is in the print function.
Each queryset contains a cache to minimize database access:
- The first time the queryset is evaluated (the first time we hit the database with that queryset), the cache is empty; after that, Django saves the result.
- If the queryset is again evaluated, the cache will be used instead of hitting the database.
Django provides an explain function that shows the execution plan of the queryset:
CODE: https://gist.github.com/brunomichetti/ec58553fc6c14e8d8846bd1508c5e732.js
This is useful when you want to analyze queries that you may need to optimize.
Best practices for using Querysets
The following is a list of good practices to take into consideration to be efficient when using querysets:
- Try to reduce the number of hits to the database as much as possible.
- When working on data from queries, try to make the database do as much work as possible instead of handling that work yourself with python code (sorting, comparing fields, etc.).
- If you know what fields you will need, try to fetch only those fields instead of all of them for each instance.
- Don’t trigger hits to the database if it’s not necessary (for example, to get an empty queryset).
- Create a database index for any unique field you know you will frequently use to search instances.
- If you are working not only with a particular model instance but also with instances of models related to it, see if you can use [.c-inline-code]select_related[.c-inline-code] and [.c-inline-code]prefetch_related[.c-inline-code] to improve your queries.
- When you have an instance of a given model A in memory, and you want to access the id of a related model instance (let’s call it model B), use [.c-inline-code]instance_a.instance_b_id[.c-inline-code] instead of [.c-inline-code]instance_a.instance_b.id[.c-inline-code]. Why? When you define a foreign key from A to B, you already have the id of the model B instance in the model A table as an attribute. In the next section, we will explain this a little bit more.
- For updating/creating multiple instances of a Model, it’s better to do it as a bulk operation by using [.c-inline-code]bulk_update[.c-inline-code] or [.c-inline-code]bulk_create[.c-inline-code], respectively, instead of one by one. Consider that using these functions doesn’t trigger the save or create methods, so any custom code in those methods will not be executed.
Next, we will show a defined reality and introduce examples for all concepts. After that, we will go into more detail regarding best practices.
Reality example
Our reality example here is the Target app:
- Users register in the system with their email.
- They also have optional data: name, username, date of birth, and zodiac sign.
- They can create/update at most ten targets on an actual map.
- Each target has a title, topic, radius in meters, and location.
- When a user creates a target in the map in a given location with a given radius, the system checks if there are other targets from other users that have the same topic and intersecting area. If that happens, a match is created between those owner users. The app then allows them to know each other.
- Each user has a list of matched users (that user has at least one match with him).
The following is an example image of matching targets with the intersecting area and the same topic:
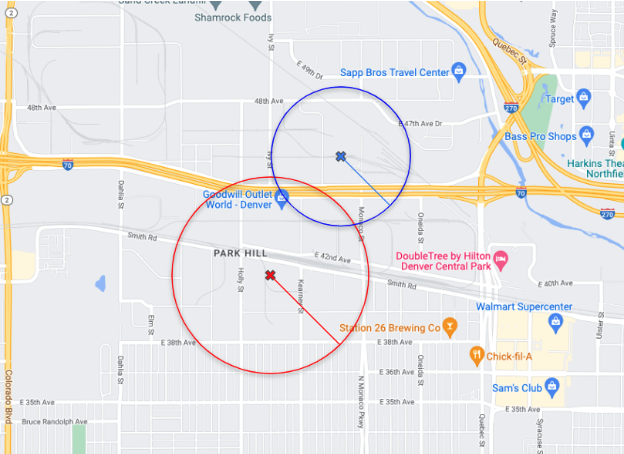
Models and relationships
The following is a simple diagram showing the models and relationships:
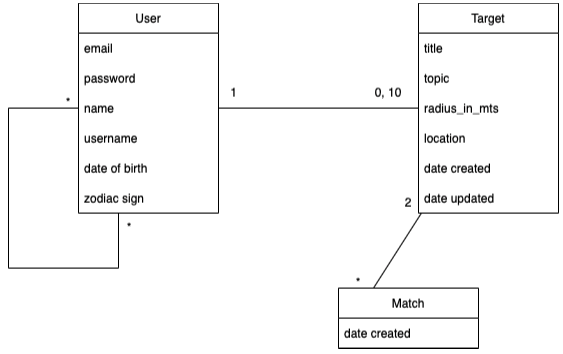
Models definition
Here are the models defined from reality example:
CODE: https://gist.github.com/brunomichetti/b7367342a75620d03308d6fca6f75499.js
Examples of good practices
Seeing SQL statement/s triggered by a queryset
To see what SQL query is going to be executed for a particular Django queryset you have two options (both require you to launch a python console using [.c-inline-code]python manage.py shell[.c-inline-code] or [.c-inline-code]python manage.py shell_plus[.c-inline-code] if you have the django-extensions library installed):
Option 1
You can use either [.c-inline-code]print(queryset.query)[.c-inline-code] or [.c-inline-code]str(queryset.query)[.c-inline-code]:
CODE: https://gist.github.com/brunomichetti/d0e4787cffa36a8233188f20508cbec6.js
Option 2 (the Django DEBUG setting must be set to True)
You can use the queries attribute of the connection module from [.c-inline-code]django.db[.c-inline-code]:
CODE: https://gist.github.com/brunomichetti/1ca76c938ca8447a0b1a7442153bcd24.js
connection.queries will display a list of dictionaries with the following keys:
- sql, whose value is the SQL statement
- time, whose value is how long the statement took to execute (in seconds)
Example:
CODE: https://gist.github.com/brunomichetti/0b32a0f9701da575823d66d1dda977e8.js
All queries executed since the start of the session will be listed; it doesn't matter when the connection module was imported. If at any point you want to clear the queries list, you can use the [.c-inline-code]reset_queries[.c-inline-code] function:
CODE: https://gist.github.com/brunomichetti/c3831f57111a23ca9d786712300bdc16.js
Using these tools, you can also count how many hits to the database a particular queryset triggers. You can do this by looking at the difference in the value of [.c-inline-code]len(connection. queries)[.c-inline-code] before and after the queryset is executed, or by clearing the queryset list and then looking at the importance of [.c-inline-code]len(connection.queries)[.c-inline-code] after evaluating the queryset.
Count vs len
As a rule of thumb, if you only want to know how many elements are in a queryset, it's best to use the queryset count function instead of using [.c-inline-code]len(queryset)[.c-inline-code], except if the queryset was already evaluated. In that case, the count will return the len of the queryset's cached value).
For example, let's try to fetch how many users we have in our database:
CODE: https://gist.github.com/brunomichetti/716458c29b18656015c40106fbeea4e9.js
As you can see, using [.c-inline-code]len(queryset)[.c-inline-code] first evaluates the queryset, so it fetches all the users and then calculates the length of the collection.
In contrast, when using the count function, the users are counted at the database level, which is more efficient memory-wise since you avoid every user instance being loaded into memory and is also generally faster.
Fetching only necessary fields
Sometimes, you may want to use only a specific subset of fields from a model. In those cases, it may be a good idea to only get those fields for every row in the database, so you avoid fetching and loading into memory data that you will not use.
As how there are many options. Using our previous example with the user model, let’s say you want to retrieve only the id and email fields; you could use:
- [.c-inline-code]User.objects.values("id", "email")[.c-inline-code]
- [.c-inline-code]User.objects.only("id", "email")[.c-inline-code]
- [.c-inline-code]User.objects.values_list("id", "email")[.c-inline-code]
CODE: https://gist.github.com/brunomichetti/148f77deadf46b040bc2457f8919030b.js
As you can see, all the options presented execute the same SQL statement under the hood. The difference is that one returns a list of dictionaries and the other a list of tuples, with the last one a list of model instances.
It’s worth noting that you can still access fields other than id and email in the instances fetched with only, but a different SQL query will be triggered specifically to fetch the value of that field if you do so.
In case you want to select all the fields except a minor subset of them, you can use defer.
Empty queryset without hitting the database
In some specific cases, you could need an empty queryset, and a natural solution for this would be to make a queryset using a filter with the condition that no model instances could meet.
This would not be the best way since you could still hit the database at least once to evaluate the queryset.
The best way would be using the querysets none function, which provides you with an empty queryset without triggering any database hits. But, if you need to use a filter to generate your empty queryset, you can use the condition [.c-inline-code]pk__in=[][.c-inline-code], any model instance can’t meet this condition, and the ORM is smart enough to realize this and not make any hits to the database.
CODE: https://gist.github.com/brunomichetti/63f82180397af98d21a3cb550113c068.js
Access the id of a related model instance
When a model A has a [.c-inline-code]ForeignKey[.c-inline-code] field to a model B, every instance of A has a field called [.c-inline-code]b_id[.c-inline-code] (name derived from the name of the related model in snake case + the word “id”).
So, when we fetch an instance of A from the database we already have the id of its related B instance available. One can wonder if there is a difference then between using [.c-inline-code]a.b_id[.c-inline-code] and [.c-inline-code]a.b.id[.c-inline-code].
Let’s try with an example from our reality:
CODE: https://gist.github.com/brunomichetti/e60f08b32b6062f47fd82d39a66f9f64.js
As we can see, when accessing the user id through [.c-inline-code]target.user_id[.c-inline-code], we trigger no SQL query since this value is part of the data we fetched from the database when we retrieved our target instance. But, when accessing target.user.id, we trigger a new SQL query to retrieve every field of the related user (unless you use the [.c-inline-code]select_related[.c-inline-code] function).
Bulk operations
As mentioned before, when working with several instances, it’s better to use bulk operations to reduce the number of hits to the database.
As an example, if we want to create several users, we can do the following:
CODE: https://gist.github.com/brunomichetti/c78a51fe3156340f7acfebd940150522.js
This works but we are hitting the database 3 times. With [.c-inline-code]bulk_create[.c-inline-code], we can do the very same thing hitting only once, defining a list of objects:
CODE: https://gist.github.com/brunomichetti/64309b581b4960045e7e8a424d304e44.js
In the same way, we can use [.c-inline-code]bulk_update[.c-inline-code], but in this case, we must have a list of user objects already created in the database. Suppose we want this for each user that has a name but doesn’t have a username:
- Get the name, remove spaces, and transform it to lowercase. This value will be the username.
- As an example, if the user’s name is John Doe, then we want the username to be johndoe.
This is a solution to get this done:
CODE: https://gist.github.com/brunomichetti/41625ce7dd2f481dacc5bf771563c130.js
The [.c-inline-code]bulk_update[.c-inline-code] receives two lists:
- The list of modified objects that you want to update. These objects already exist in the database and you have changed at least one field.
- The list of columns that you have changed in the objects.
The corresponding SQL query:
CODE: https://gist.github.com/brunomichetti/7468793c5c9056cfd4e88b89a84f40b1.js
Some important considerations:
- When using [.c-inline-code]bulk_create[.c-inline-code] and [.c-inline-code]bulk_update[.c-inline-code], the model’s save function is not executed.
- The [.c-inline-code]bulk_create[.c-inline-code] function:
- doesn’t work with many-to-many relationships.
- doesn’t work with child models in a multi-table inheritance scenario.
- accepts a [.c-inline-code]batch_size[.c-inline-code] param in case the list of objects to create is too big. This param splits the bulk creation in more hits to the database but helps to avoid very big SQL queries.
- The [.c-inline-code]bulk_update[.c-inline-code] function:
- can’t update the primary key field.
- if updates fields defined on multi-table inheritance ancestors will generate an extra query per ancestor.
- if updates a large number of columns in a large number of rows, the SQL generated can be very large. Avoid this by adding a suitable [.c-inline-code]batch_size[.c-inline-code] param to the function. This is analogous to [.c-inline-code]batch_size[.c-inline-code] in [.c-inline-code]bulk_create[.c-inline-code]
Querysets examples
In this section, we will describe different queries examples to show efficient ways of addressing them. We will describe each case in natural language, then present the queryset and finally show the resultant SQL query.
F expressions
As mentioned, if possible it's recommended to delegate computations to the database instead of doing them directly in memory.
F expressions help us to do precisely that, allowing us to reference database fields and letting the database handle operations with that value.
For example in the following query:
CODE: https://gist.github.com/brunomichetti/a2ccc33622364da2d3582e683ae31b1e.js
Filters all targets that have an updated_at value posterior to their created_at value, but the value of updated_at is never loaded into memory, it's just referenced so the database can use it in the comparison:
As we can see in the generated SQL:
CODE: https://gist.github.com/brunomichetti/2c85b93b8725a40e2a4b37217203ee70.js
Another use of F expressions can be for updating database rows. For example, if we wanted to increase the [.c-inline-code]created_at[.c-inline-code] value for all rows by 3 days, we could retrieve all instances, modify the attribute value, and save all instances.
This would require at least 2 hits to the database, but using F expressions we can do it in just one:
CODE: https://gist.github.com/brunomichetti/b943aac74e0163494c0af9df1903f0a6.js
This generates the following SQL query:
CODE: https://gist.github.com/brunomichetti/d06d8d6c7bfc2fb09a0828f25226dde8.js
Q objects
Q objects represent conditions that can be used in database queries. They are helpful not only because they let you reuse conditions in multiple questions but also because they can be combined to express complex conditions.
A common use case for Q objects is defining an OR condition for a filter. As an example, let’s retrieve the targets whose topic is “Sports” or that contains the word sport in their title:
CODE: https://gist.github.com/JorgeMichelena/9a0f02c9a9c8f54b111b6bf4361064d8.js
The generated SQL is as follows:
CODE: https://gist.github.com/JorgeMichelena/321643c644b2028b3787d769f4f0f31c.js
N+1 problem
Imagine if we need to print for each target: the target title, and then the email of the corresponding owner user. This can be a solution:
CODE: https://gist.github.com/JorgeMichelena/1516885d92552ea93d49d7236120fd9b.js
This is a working solution, but how many times does it access the database? Imagine that N is the number of targets in the system:
- Accesses one time to get all the targets ([.c-inline-code]Target.objects.all() : 1[.c-inline-code])
- For each target accesses one time to get the email of the owner user ([.c-inline-code]target.user.email : N[.c-inline-code])
- To get the title doesn’t need to access the database because it’s brought in the first query.
That is a total of N + 1 hits. As mentioned, it’s important to reduce the number of hits to the database. If N is a huge number, then we are accessing it a lot of times and this will generate a performance issue. This is known as the N + 1 problem.
Let’s look at a way of addressing this with [.c-inline-code]select_related[.c-inline-code] and [.c-inline-code]prefetch_related[.c-inline-code].
Select related
Let’s see the definition in Django docs:
This returns a [.c-inline-code]QuerySet[.c-inline-code] that will “follow” foreign-key relationships, selecting additional related-object data when it executes its query. This performance booster results in a single, more complex question but means later use of foreign-key relationships won’t require database queries.
Continuing the example, the solution would be this:
CODE: https://gist.github.com/JorgeMichelena/0f32ad5f7fde61ddc6a0b3757a75bbc1.js
Now, the output is the same as before, but we only access the database once with this solution. That is because [.c-inline-code]select_related[.c-inline-code] brought in the same query, each user's email. We can use [.c-inline-code]selected_related[.c-inline-code] with foreign-key and one-to-one relationships fields. Let’s see now how to do this when we have many-to-many relationships.
Prefetch related
Now we need to print for each user: the user id and the emails of the related users that matched with him (remember we have the [.c-inline-code]mateched_users[.c-inline-code] relationship). Let’s see the definition in the Django docs:
This returns a [.c-inline-code]QuerySet[.c-inline-code] that will automatically retrieve, in a single batch, related objects for each of the specified lookups.
So, it has the same purpose as [.c-inline-code]select_related[.c-inline-code] but it has a different strategy. The solution to the mentioned problem using the function is this:
CODE: https://gist.github.com/JorgeMichelena/8fbcbe4eefa6cecd3d53cd9475cba219.js
This function accesses two times to the database:
- One time to get all the users ([.c-inline-code]User.objects.all() : 1[.c-inline-code]).
- A second time to get the matched users of each user ([.c-inline-code].prefetch_related("matched_users") : 1[.c-inline-code]).
If we didn't use the [.c-inline-code]prefetch_related[.c-inline-code] function in the previous solution, we would have the database accessed N + 1 times (being N the number of users in the system).
Quick summary:
- select_related solves the N + 1 problem accessing only one time to the database. It’s used for foreign-key and one-to-one relationships fields.
- prefetch_related solves the N + 1 problem accessing only two times to the database. It’s used for many-to-many relationships fields.